网站首页 > 技术文章 正文
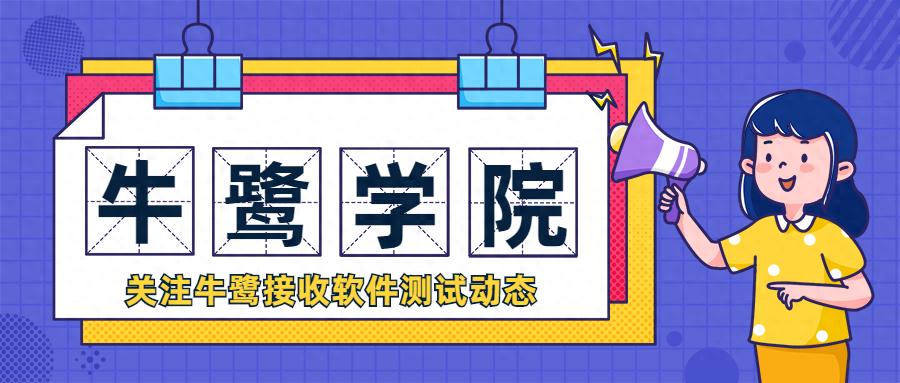
1. 构建Series对象
def build_series():
"""
使用pandas创建Series对象(一维的数组型对象)
:return:
"""
# 创建数组
pd.Series(dict(names='Evan', id=66)) # 使用字典生成一个Series(字典的键是行索引)
test = pd.Series(['aa', 'bb', 'cc', 'aa'], index=[1, 3, 5, 7]) # 使用列表生成一个Series,并指定行索引值(默认从0开始)
test.name = 'Data' # 指定数组名称
test.index.name = 'Info' # 指定行索引名称
# 查看数组属性
print('返回数组名称\n{}'.format(test.name))
print('返回指定行索引内的值\n{}'.format(test[3]))
print('返回数组的所有值\n{}'.format(test.values))
print('返回数组的所有索引\n{}'.format(test.index))
print('返回数组所有的唯一值(去重)\n{}'.format(test.unique()))
print('返回数组所有值的出现次数(默认降序)\n{}'.format(test.value_counts()))
print('计算数组中每个值是否包含于传入序列的值,返回一个布尔值\n{}'.format(test.isin(['aa', 'cc'])))
2. 构建DataFrame对象
def build_data_frame():
"""
使用pandas创建DataFrame对象(二维的数组型对象)
:return:
"""
# 创建数组
# 1. 使用嵌套字典格式创建DataFrame(字典的键作为列标签,内部字典的键作为行索引)
data = {
'Name': {'Evan': 1, 'Jane': 2},
'Id': {'Evan': 11, 'Jane': 22}
}
print('使用嵌套字典构建DataFrame\n{}'.format(pd.DataFrame(data)))
# 2. 使用字典列表格式创建DataFrame(字典的键为列标签,行索引默认从0开始,列表内的所有值是每一列的值)
data = {
'Name': ['Evan', 'Jane', 'Spring', 'Summer', 'Autumn', 'Winter'],
'Id': [66, 77, 88, 99, 100, 110],
'Stature': [177, 160, 150, 167, 180, 190],
'Weight': [65, 45, 50, 60, 65, 70]
}
# 指定行索引值、列标签顺序(使用columns参数时,只会读取该列表内的列索引数据)
frame = pd.DataFrame(data, columns=['Id', 'Name', 'Weight', 'Stature'], index=[3, 2, 1, 6, 5, 4])
print('构建指定列标签顺序和行索引的DataFrame\n{}'.format(frame))
# 查看数组属性
print('返回数组的行索引是否唯一\n{}'.format(frame.index.is_unique))
print('返回数组的头5行\n{}'.format(frame.head()))
print('返回指定行索引内的指定列标签值(使用轴标签)\n{}'.format(frame.loc[2, ['Id', 'Weight']])) # 使用行索引和列标签的值
print('返回指定行索引内的指定列标签值(使用整数标签)\n{}'.format(frame.iloc[1, [0, 2]])) # 使用行索引和列标签的位置
print('返回指定行索引内的所有值\n{}'.format(frame.loc[2]))
print('返回指定列标签内的所有值\n{}'.format(frame['Name']))
print('返回数组所有的列标签\n{}'.format(frame.columns))
print('返回数组所有的值\n{}'.format(frame.values))
print('返回数组中所有值在每一列的出现次数(没有的默认为0)\n{}'.format(frame.apply(pd.value_counts).fillna(0)))
print('返回数组中指定列标签内所有值的出现次数\n{}'.format(pd.value_counts(frame['Weight'])))
print('返回数组中指定列标签内所有的唯一值(去重)\n{}'.format(pd.unique(frame['Weight'])))
3.数组操作
def array_operations():
"""
数组操作
:return:
"""
data = {
'Name': ['Evan', 'Jane', 'Spring', 'Summer', 'Autumn', 'Winter'],
'Id': [66, 77, 88, 99, 100, 110],
'Stature': [177, 160, 150, 167, 180, 190],
'Weight': [65, 45, 50, 60, 65, 70]
}
frame = pd.DataFrame(data, columns=['Id', 'Name', 'Weight', 'Stature'], index=[3, 2, 1, 6, 5, 4])
# 对数组排序
# inplace默认False,代表是否改变原数组,为True时返回None,False返回一个新数组
print('根据行索引排序(默认升序)\n{}'.format(frame.sort_index(inplace=True)))
print('根据行索引排序(降序)\n{}'.format(frame.sort_index(ascending=False))) # 指定降序
print('根据列标签排序\n{}'.format(frame.sort_index(axis='columns')))
data = pd.Series([3, 6, -5, 8, 3])
print('根据数组值排序\n{}'.format(data.sort_values()))
print('根据指定列标签排序\n{}'.format(frame.sort_values(by=['Weight', 'Stature'])))
# 对数组排名
print('对所有值进行排名(默认升序)\n{}'.format(data.rank())) # 默认升序排名,平级全部取平均值
print('对所有值进行排名(降序)\n{}'.format(data.rank(ascending=False))) # 降序排名,平级全部取平均值
print('平级关系取最小值,占用坑位\n{}'.format(data.rank(method='min'))) # 平级关系全部取最小值,每个平级关系占用一个坑位
print('平级关系取最小值,不占用坑位\n{}'.format(data.rank(method='dense'))) # 平级关系全部取最小值,平级关系不占用坑位
print('平级关系取最大值,占用坑位\n{}'.format(data.rank(method='max'))) # 平级关系全部取最大值,每个平级关系占用一个坑位
print('按观察顺序排名\n{}'.format(data.rank(method='first'))) # 按照值在数据中出现的次序分配排名
# 重构数组
# ffill向前填充(缺失值和其前面的值相同),bfill向后填充(缺失值和其后面的值相同)
print('重新构建数组的行索引\n{}'.format(frame.reindex([1, 3, 5, 7], method='ffill')))
# fill_value赋给缺失值
print('重新构建数组的行索引\n{}'.format(frame.reindex([2, 4, 6, 8], fill_value='world')))
print('重新构建数组的列标签\n{}'.format(frame.reindex(columns=['Id', 'Weight', 'happy'], fill_value='news')))
# 删除指定的项目
print('删除指定行\n{}'.format(frame.drop([1, 3])))
print('删除指定列\n{}'.format(frame.drop(['Id', 'Weight'], axis='columns'))) # axis控制轴的位置,默认为index
4.文件操作
def file_operations():
"""
使用pandas载入存储文件
:return:
"""
data = pd.DataFrame([[1, 2, 3, '11'], [4, 5, 6, '22'], [7, 8, 9, None]], columns=['a', 'b', 'c', 'd'])
# 写入读取CSV文件
file_name = 'example.csv'
# index:是否写入行索引,header:是否写入列标签
# columns:可指定写入对应的列标签数据并且有顺序,na_rep会赋值给缺失值(默认为空)
data.to_csv(file_name, index=False, header=False, columns=['b', 'd', 'a'], na_rep='NULL') # 写入CSV文件
data.to_csv(sys.stdout, sep='|') # 输出到屏幕,sep为分隔符(可用于临时查看写入的数据)
print('读取CSV文件')
print('自动分配默认列标签(从0开始)\n{}'.format(pd.read_csv(file_name, header=None)))
print('指定列标签\n{}'.format(pd.read_csv(file_name, names=['a', 'b', 'c'])))
print('指定"a"列的值为行索引\n{}'.format(pd.read_csv(file_name, names=['a', 'b', 'c'], index_col='a')))
print('指定"a"列和"b"列的值为行索引(分层索引)\n{}'.format(pd.read_csv(file_name, names=['a', 'b', 'c'], index_col=['a', 'b'])))
print('使用缺失值替换文件内的指定值\n{}'.format(pd.read_csv(file_name, names=['a', 'b', 'c'], na_values={'b': [5, 8]})))
# pd.options.display.max_rows = 5 # 设置文件读取显示的行数,多出的行数变为省略号显示
print('跳过指定的行读取\n{}'.format(pd.read_csv(file_name, names=['a', 'b', 'c'], skiprows=[0, 2]))) # 跳过第0行和第2行
print('读取指定的行范围\n{}'.format(pd.read_csv(file_name, names=['a', 'b', 'c'], nrows=2))) # 只读取前2行
os.remove(file_name)
# 写入读取Excel文件
file_name = 'example.xls'
data.to_excel(file_name, index=True, header=True, sheet_name='sheet1', na_rep='NULL')
print('读取Excel文件')
print(pd.read_excel(file_name, sheet_name='sheet1'))
os.remove(file_name)
# 写入读取JSON文件
file_name = 'example.json'
data.to_json(file_name)
print('读取JSON文件')
print(pd.read_json(file_name))
os.remove(file_name)
# 读取TXT文件
print('读取TXT文件')
file_name = 'example.txt'
with open(file_name, 'w', encoding='utf-8') as f:
f.writelines(['A,B,C\n', '1,2,3\n', '4,5,6\n', '4,5,6\n'])
print(pd.read_csv(file_name, sep=',')) # 使用sep根据逗号切分每个数据
os.remove(file_name)
5.数据清洗
def data_cleansing():
"""
数据清洗
:return:
"""
nan = np.NAN
# 过滤缺失值
data = pd.DataFrame([[1, 2, 3], [4, 5, nan], [None, nan, nan], [1, 2, 3]], columns=['a', 'b', 'c'])
print('过滤所有包含缺失值的行\n{}'.format(data.dropna())) # 默认删除包含缺失值的行
print('过滤所有包含缺失值的列\n{}'.format(data.dropna(axis='columns')))
print('过滤所有值均为NA的行\n{}'.format(data.dropna(how='all'))) # 只删除所有值均为NA的行
print('过滤并保留包含一定数量NA的行\n{}'.format(data.dropna(thresh=1))) # 保留一行NA值
# 补全缺失值
print('补全所有缺失值\n{}'.format(data.fillna(0))) # 全部的缺失值变为0
print('只补全指定列标签内的缺失值\n{}'.format(data.fillna({'a': 0, 'b': 1}))) # 只补全"a"列和"b"列的缺失值
print('补全所有缺失值(向前填充)\n{}'.format(data.fillna(method='ffill', limit=1))) # 向前填充(缺失值和其前面的值相同),limit为最大填充范围
print('补全所有缺失值(向后填充)\n{}'.format(data.fillna(method='bfill'))) # 向后填充(缺失值和其后面的值相同)
# 删除重复值
print('删除所有重复的行(默认保留第一个观测到的行)\n{}'.format(data.drop_duplicates()))
print('删除所有重复的行(保留最后一个观测到的行)\n{}'.format(data.drop_duplicates(keep='last')))
print('删除指定列标签下重复的行\n{}'.format(data.drop_duplicates(['b'])))
# 替代值
print('替换所有的NA为0\n{}'.format(data.replace(nan, 0)))
print('替换指定数量的值\n{}'.format(data.replace({1: 10, 2: 20}))) # 1替换为10,2替换为20
# 重命名轴索引
print('重命名行索引\n{}'.format(data.rename(index={1: 10})))
print('重命名列标签\n{}'.format(data.rename(columns={'c': 'cc'})))
6.数据规整
def data_structured():
"""
数据规整
:return:
"""
# Series分层索引
data = pd.Series(np.random.randn(6), index=[['a', 'a', 'b', 'b', 'c', 'c'], [1, 2, 3, 1, 2, 3]])
print('创建Series分层索引\n{}'.format(data))
print('分层索引重新排列\n{}'.format(data.unstack())) # 第二索引变为列标签(Series对象转化为DataFrame对象)
print('分层索引复原\n{}'.format(data.unstack().stack()))
# DataFrame分层索引
data = pd.DataFrame(np.arange(12).reshape((4, 3)), index=[['a', 'a', 'b', 'b'], [1, 2, 1, 2]],
columns=[['one', 'one', 'two'], ['three', 'four', 'five']])
# 定义层级行名称和列名称
data.index.names = ['key1', 'key2']
data.columns.names = ['number1', 'number2']
print('创建DataFrame分层索引\n{}'.format(data))
# 层级交换(数据不会跟着变)
print('行层级名称交换(使用名称)\n{}'.format(data.swaplevel('key1', 'key2')))
print('列层级名称交换(使用序号)\n{}'.format(data.swaplevel(0, 1, axis='columns')))
# 层级排序(数据会跟着变)
print('行层级名称排序\n{}'.format(data.sort_index(level=1)))
print('列层级名称排序\n{}'.format(data.sort_index(level=1, axis='columns')))
# 按层级进行汇总统计
print('按行层级求和\n{}'.format(data.sum(level='key1')))
print('按列层级求和\n{}'.format(data.sum(level='number2', axis='columns')))
# 将DataFrame的列变为行索引
data = pd.DataFrame(np.arange(12).reshape((4, 3)), columns=['a', 'b', 'c'])
print('将DataFrame的列变为行索引(移除该列)\n{}'.format(data.set_index(['c']))) # "c"会从数组中移除变为行索引
print('将DataFrame的列变为行索引(保留该列)\n{}'.format(data.set_index(['c'], drop=False))) # "c"会保留在数组中
猜你喜欢
- 2025-01-05 译|Python幕后(3):漫步CPython源码
- 2025-01-05 c语言和python的区别
- 2025-01-05 Python常见的数据结构实现
- 2025-01-05 为什么我的python总是学不好
- 2025-01-05 #!/usr/bin/python与#!/usr/bin/env python的区别
- 2025-01-05 Python爬虫入门之爬取图片
- 2025-01-05 python封装使用语法规则
- 2025-01-05 一张思维导图概括Python的基本语法, 一周的学习成果都在里面了
- 2025-01-05 妙趣横生Python海龟图turtle
- 2025-01-05 使用Python获取HTTP请求头数据
- 05-25Python 3.14 t-string 要来了,它与 f-string 有何不同?
- 05-25Python基础元素语法总结
- 05-25Python中的变量是什么东西?
- 05-25新手常见的python报错及解决方案
- 05-2511-Python变量
- 05-2510个每个人都是需要知道Python问题
- 05-25Python编程:轻松掌握函数定义、类型及其参数传递方式
- 05-25Python基础语法
- 257℃Python短文,Python中的嵌套条件语句(六)
- 257℃python笔记:for循环嵌套。end=""的作用,图形打印
- 256℃PythonNet:实现Python与.Net代码相互调用!
- 251℃Python操作Sqlserver数据库(多库同时异步执行:增删改查)
- 251℃Python实现字符串小写转大写并写入文件
- 106℃原来2025是完美的平方年,一起探索六种平方的算吧
- 91℃Python 和 JavaScript 终于联姻了!PythonMonkey 要火?
- 81℃Ollama v0.4.5-v0.4.7 更新集合:Ollama Python 库改进、新模型支持
- 最近发表
- 标签列表
-
- python中类 (31)
- python 迭代 (34)
- python 小写 (35)
- python怎么输出 (33)
- python 日志 (35)
- python语音 (31)
- python 工程师 (34)
- python3 安装 (31)
- python音乐 (31)
- 安卓 python (32)
- python 小游戏 (32)
- python 安卓 (31)
- python聚类 (34)
- python向量 (31)
- python大全 (31)
- python次方 (33)
- python桌面 (32)
- python总结 (34)
- python浏览器 (32)
- python 请求 (32)
- python 前端 (32)
- python验证码 (33)
- python 题目 (32)
- python 文件写 (33)
- python中的用法 (32)